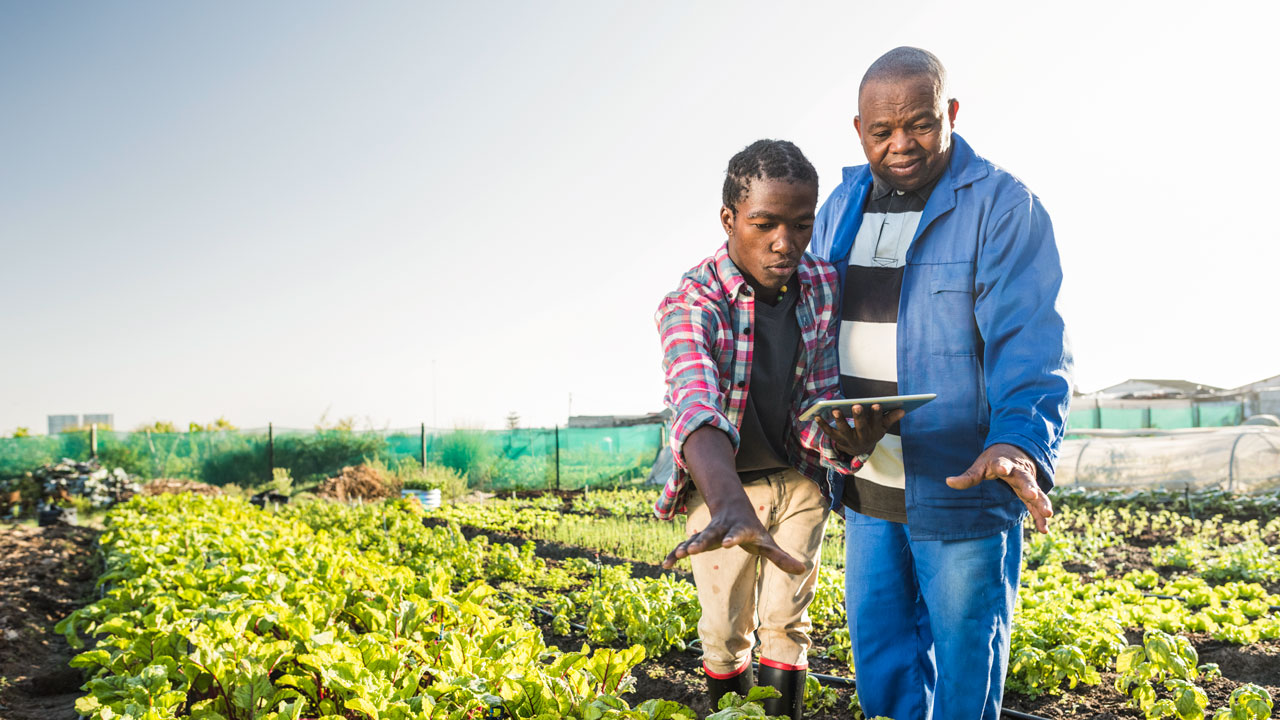
This article was originally published on Agrilinks.
Recent advances in earth observation technologies, coupled with advanced artificial intelligence and machine learning analytics, hold significant potential to help achieve food security outcomes at scale. The widespread use of more accurate, timely, and applicable crop analytics is a key step in improving agricultural production and systemic resilience, including those smallholder systems which are highly sensitive to changing weather patterns and climate change. With the right information, advanced analytic providers can help tailor advice and insurance or other products to the hyperlocal level, driven by the unique agroclimatic conditions of a given farm. When paired with effective extension and outreach, farmers can make more informed farm management decisions within a current season (e.g., timing of spraying, planting, and harvesting), or better understand the types and varieties of crops that may thrive based on their specific and local agroecological zones.
To realize the potential of AI and ML, crop analytic providers require training and validation or ground truth data to match the conditions on the ground at a given time and place with corresponding earth observation imagery. Researchers, advanced analytics providers, and field-based project implementers have continued to better coordinate efforts to align data collection and sharing efforts. Yet, often the agricultural datasets generated by those operating in the field lack the geospatial or temporal characteristics that make the data usable in ML applications. Despite recent gains in developed markets, there is not enough high-quality, timely, and interoperable ground truth data in smallholder agriculture production systems to realize the potential benefits of advanced crop analytics. This is especially true in smallholder markets, which often are dominated by heterogenous cropping patterns, the return on investment in data collection and analytic costs are more difficult to achieve.
To help close the data gap and meet the needs of the analytics community, Tetra Tech is implementing the Enabling Crop Analytics at Scale (ECAAS) initiative, funded by the Bill & Melinda Gates Foundation. This three-year effort was designed to catalyze the development, availability, and uptake of agricultural remote-sensing data and subsequent applications in smallholder farming systems. Specific program objectives include:
- Develop and test innovative strategies and methods to reduce costs of collecting ground-truth data or further its use within the crop analytics data chain
- Identify, map, and promote standards for collecting and aggregating high-quality training data, driven by priority end-use case applications, including:
- On-farm extension and production
- Food security, early warning, and related decision support systems
- Smallholder access improvements to tailored debt and insurance products
- Facilitate the scaling of training dataset creation and exchange through an improved data-sharing ecosystem and more cohesive analytics community
- Improve access to repositories of regularly updated ground data and core insight layers (e.g., field boundary identification, crop type identification, and yield estimates) to enable scalable, satellite-based analytics in emerging agricultural economies
In support of these objectives, Tetra Tech partnered with the Radiant Earth Foundation, Get ODK Inc., and D4DInsights to develop and launch the ECAAS Field Mapper for ODK. This free-to-use mobile data collection toolkit helps organizations more easily collect ML-ready data in a standard catalog compliant with SpatioTemporal Asset Catalog specifications. Should the data collector choose to do so, datasets can automatically be uploaded to the Radiant Earth ML Hub, processed and cleaned as needed, and then accessed by the community. By combining standardized data formatting with improved accessibility, this system can help significantly decrease the difficulty and cost of developing and deploying advanced crop analytics applications, which rely on fundamental agricultural parameters (e.g., field boundary, crop type, and yield).
The ECAAS team has designed Field Mapper through consultations with more than 15 organizations, including World Food Programme, Clark University, One Acre Fund, McKinsey & Company, and others. The toolkit includes a tutorial video for those unfamiliar with ODK Collect, as well as access to a community help desk. The ECAAS team encourages interested parties to check out the form, get in touch, and, more importantly, begin deploying the toolkit within agricultural data capture or monitoring and evaluation efforts. With every additional dataset captured through this process, the ML-ready ground truth data lake deepens. Combining these data with the latest in earth observation technology within an improved data-sharing ecosystem significantly increases the potential for more accurate, timely, and useful crop analytics, improving agricultural production and food security outcomes.
About the authors
Tetra Tech's Agriculture and Economic Growth team
Tetra Tech’s experts focused on infusing technology into agriculture, food security, and economic growth projects worldwide. Learn more about the team and their services in agriculture and food security.