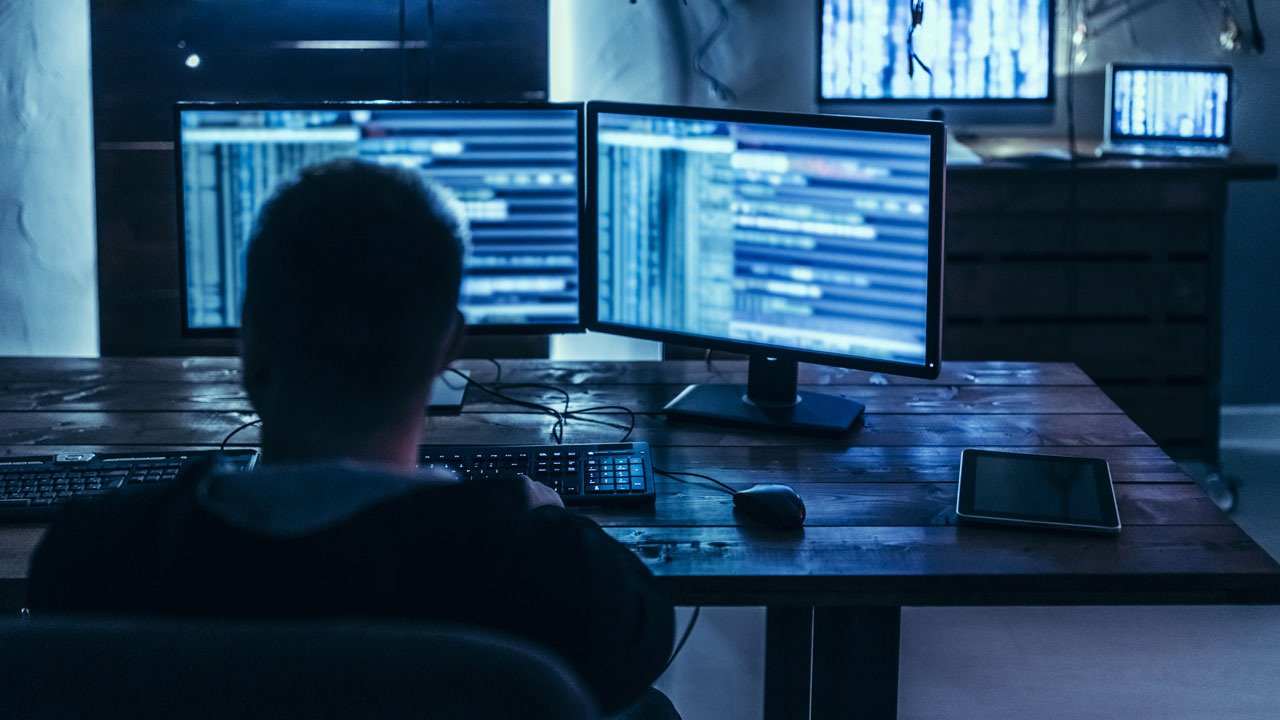
The challenge becomes more complex as enterprise attack surfaces continue to grow. For example, organizations are continually adding more fixed and mobile devices, which can reach into the hundreds of thousands of people, depending upon the size of the organization. These devices, in turn, collect and store more and more sensitive and proprietary data that needs to be protected. The result is a rise in attack vectors—the means by which a hacker can gain access to a computer or network server. This also means maintaining an organization’s security posture is gradually exceeding the capacity of humans alone.
Artificial intelligence (AI) combined with human knowledge is the answer to solving this challenge. With machine learning (ML), a subset of AI, algorithms can rapidly assess high volumes of security events and identify potential threats, ranging from malware to high-risk human behavior. These algorithms learn and adapt over time, using past data and patterns to identify new attacks and gradually becoming more autonomous. AI can keep pace with bad cyber actors and respond more effectively and adaptively than conventional rules-driven approaches.
The success of using AI and ML to respond to cyberattacks depends on the data available to train the algorithms. At its core, ML is about identifying patterns, which can only be as comprehensive as the data used. For cyber defense this means that it is critical to gather data that represents as many scenarios as possible. The data also should come from various sources, whether that is at the endpoint, on the network, or in the cloud.
Many organizations are struggling to understand the distinctions among AI; ML; robotic process automation (RPA); and security orchestration, automation, and response (SOAR)—and which technology would most benefit their security posture. The answer: it depends. Each technology has use cases that maximize its capabilities and features. Independently, each one is useful for unique use cases, but combining them can produce powerful results:
- RPA is most suited to automating well-defined and standardized business processes with expected outcomes/outputs
- SOAR detects endpoint attacks based on correlating information and security information and event management (SIEM) tools
- AI and ML add additional value because they can be integrated with the tools mentioned above to provide in-depth analysis of the available data and to automate decision-making for many cybersecurity functions
The Tetra Tech Federal IT Innovation Lab and cyber experts have been actively working to develop solutions for increasing automation in cyber defense. For example, we developed an RPA bot for security compliance that performs system security plan (SSP) validation checks in minutes. We are actively working with our customers to define new use cases for AI and related technologies and researching new ways of looking at AI and cyber approaches, such as developing a solution based on our AI chatbot, Auxilium. This solution aims to leverage natural language processing to detect social engineering threats that are commonly generated from outside the United States by non-native speakers.
Generally, the use of AI and related technologies helps minimize or mitigate the impact of cyberattacks. The benefits of implementing AI and related technologies in a cyber context include:
- Detecting threats and identifying indicators of compromise that may not be discovered using traditional cyber detection mechanisms
- Delivering consistently up-to-date knowledge of threats to help make critical prioritization decisions
- Improving understanding and ability to automate responses to cyber incidents
- Utilizing resources efficiently by focusing cybersecurity staff on high-value activities instead of repetitive tasks
- Maximizing existing investments in tools and data initiatives
- Identifying anomalous behavior proactively at all layers in context (e.g., people, assets, networks)
- Leveraging AI’s natural language capabilities to understand the origination of cyberattacks